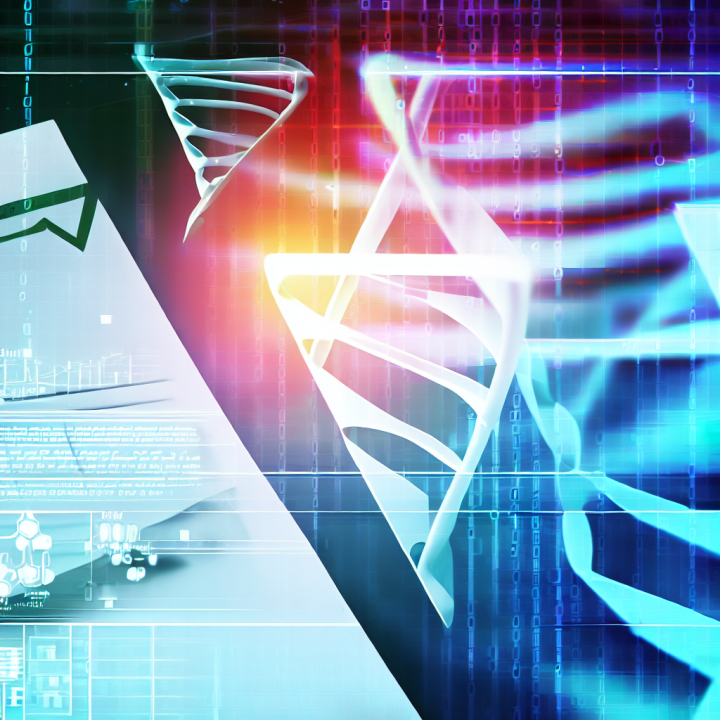
Research overview
Research in this theme is focused on combining different types of biomedical evidence in an approach often termed “triangulation”. As different types of research data have different biases, agreement between studies can help increase confidence in a finding, whilst disagreement can help identify which biases might be responsible for false positives.
Evidence extraction from the literature
Work led by PhD student Marina Vabistsevits on the identification of risk factors for breast cancer has highlighted the potential to combine literature knowledge with Mendelian randomization causal estimates. As part of our World Cancer Research Fund project led by Research Fellow Yi Liu, Senior Research Associate Maria Sobczyk-Barad and Senior Research Associate Zhaozheng Xu we are developing new approaches to extracting knowledge from the literature using large language models.
Triangulating Mendelian randomization and randomized clinical trials
In work led by Maria Sobczyk-Barad, we have systematically integrated data on clinical trials with Mendelian randomization causal estimates. This work highlighted some of the many challenges with this type of triangulation, and an ongoing project led by PhD student Winfred Gatua is focused on addressing some of the through in depth case studies of specific intervention/outcome pairs.
Triangulation of Mendelian randomization and target trial emulation
Observational data from electronic health records (EHR) can be used to emulate clinical trials in an approach called target trial emulation. In work led by Research Fellow Venexia Walker, with PhD student Si Fang and PhD student Yueying Li, we are triangulating evidence on drug repurposing and safety from target trial emulation with evidence for the corresponding drug target using MR.